Rules of Resilience: Modeling impacts of host-microbe interactions during perturbations
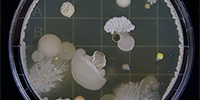
The gut microbiota has emerged as an integral component of organism well-being, influencing the most basic of functions such as metabolism, to more specialized systems e.g., immunological function. While work has been done to understand the interactions of host, microbiota, and environment in one dimension, few have attempted to integrate all three of these aspects. This gap is largely due to a lack of computational tools that have the capability to integrate and build models from the large multi-omic datasets that arise from each component. Viewing the combined data as high dimensional tensors allows us to use techniques such as factor analysis and latent feature representations to find multi-way correlations across the three components. The increased dimensionality also necessitates the need for being able to perform such analyses in a scalable fashion using modern supercomputers, (e.g., NSF-funded Frontera). We propose a new collaboration among the research teams of microbiome experimentalists and computer scientists to build a highly inter-disciplinary group with the goal of advancing knowledge (U goal 1) of how host microbe interactions respond and recover from perturbations. This work will also provide deeply engaged learning opportunities for students (U goal 2). We will collect preliminary microbiome data, and begin to design new computing approaches. This effort will be translated into a proposal to the NSF solicitation “URoL: Microbiome Theory and Mechanisms.
Current Status
2021-09-14
Abstract:
The gut microbiome has emerged as an essential component of organismal health. Current computational tools lack the ability to assess host, microbiome, and environment in an effective, comprehensive capacity. The high dimensionality of multi-omic data sets necessitate novel computational tools that are capable of utilizing modern supercomputers effectively. To address this gap in analysis techniques, we have analyzed several existing multi-omic datasets and have developed a complex dataset appropriate for these analyses. For our experimental work, we infected mice with typical and low-diversity microbiomes with parasites, and sampled animals and their microbiota before, during, and post-infection to create a high-dimensional dataset from a single, complex experiment. We created a lineage of mice with a novel, low-diversity microbial community to compare to typical mice and infected animals with the intestinal roundworm Heligmosomoides bakeri as an agent to perturb to host and microbiome. We are currently gathering data on host immune function, nutrient processing, microbiome community structure, microbiome function, and parasitic infection to yield the targeted dataset. The computational team has developed an automated software pipeline to process the data and has analyzed sample data sets to determine which combination of biological datasets we should collect. They compared AVOVA, ANOVA + VIF and a simple Autoencoder on the sample data to determine the which feature reduction technique was most effective. They also compared traditional machine learning algorithms: Logistic Regression, Support Vector Machines, Random Forest and XGBoost with deep learning algorithms:3 layer sequential neural network and a 1-D convolutional neural network, to determine which algorithms to use during the analysis phase of the project. With this work, we will generate new tools for multi-omic datasets that will be made accessible to other researchers, and we will continue to generate an understanding of the role of microbiome diversity in resistance and resilience with the new lineage of low-diversity microbiome mice that we created for this project.
Collaborators
M. Denise Dearing
College of Science
School of Biological Sciences
Project Owner
Aditya Bhaskara
College of Engineering
School of Computing
June Round
School of Medicine
Pathology
William Stephens
School of Medicine
Pathology
Hari Sundar
College of Engineering
School of Computing
Project Info
Funded Project Amount$30K
Keywords
microbiome, supercomputing, big data, environmental perturbations, metagenomics, 16S, diet, germ-free mice
Project Status
Funded 2020
Poster
View poster (pdf)